MLLMA: Multimodal Large Language Models and Applications
JANUARY 7-10, 2025 | NARA, JAPAN
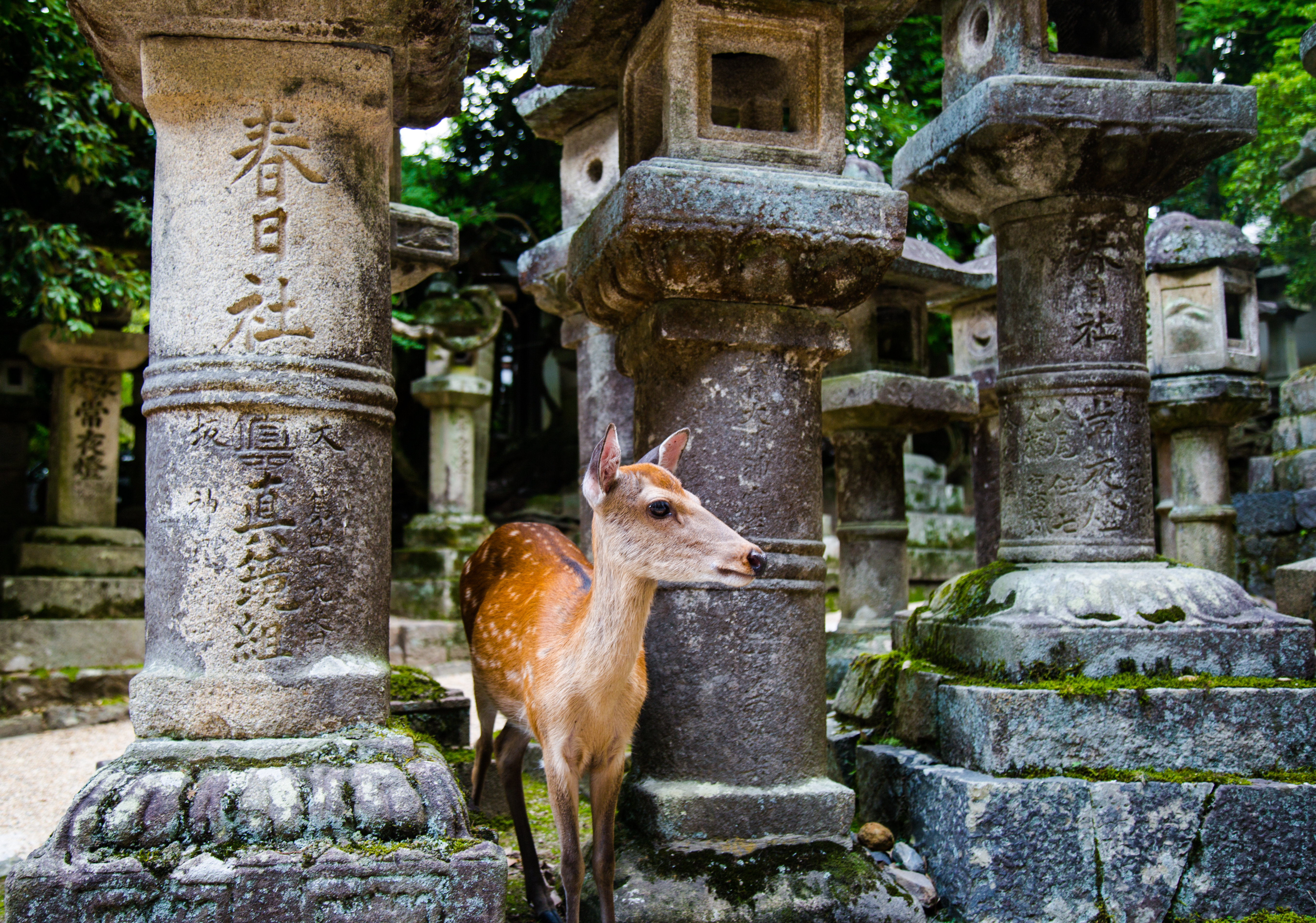
MLLMA-25 SPECIAL SESSION
ABSTRACT
The MLLMA Special Session explores the fusion of Computer Vision, NLP, and AI, focusing on cutting-edge multimodal learning techniques applied in Visual QA, Summarization, and Dialog. Its objective is to integrate multimodal analysis with large language models, bridging existing research gaps. With a theme centered on Multimodal Analysis & Large Language Models, the event highlights challenges like representation learning and QA. Through keynote speeches and paper presentations, experts engage in discussions at the forefront of this evolving field.
Objectives and Goals
Large Language Models (LLMs) are also booming in recent years as transformative entities in the realm of natural language pro- cessing, ushering in a new era of sophisticated text analysis and comprehension [10], [13]. Large Language Models (LLMs), built on advanced deep learning architectures with millions or billions of parameters, can understand and generate human-like text exceptionally well. They capture subtle language nuances and context dependencies from extensive text data through pre-training on massive datasets, gaining broad knowledge of language patterns, semantics, and syntax.Subsequently, fine-tuning on specific tasks refines their adaptability to diverse applications, from sentiment analysis and named entity recognition to language translation and summarization [1], [7], [8], [12].
The current research landscape at the intersection of multi- modality and large language models is dynamic and impactful [5], [6], [9], [11]. Researchers are actively exploring innovative ways to fuse diverse modalities, including text, images, and audio, with large language models. Key areas of focus include improving image captioning [2], sentiment analysis [3], and cross-modal retrieval [4]. Additionally, Efforts are underway to develop transparent and context-aware AI models, addressing the need for interpretability. In healthcare, multimodal approaches combine medical images, clinical notes, and patient records to enhance diagnostic tools.
The MLLMA special session aims to bring together experts from Information Retrieval, Natural Language Processing, Computer Vision, and Human Computation fields. It focuses on exploring innovative methods for analyzing multimedia data, including images, text, and videos, with a holistic approach. The goal is to develop efficient strategies for integrating large language models with multimodal data, particularly enhancing reasoning through approaches like RAG. The session welcomes contributions from academia and industry to advance research in multimodal information processing and retrieval.
IMPORTANCE, TIMELINESS AND RELEVANCE TO THE MMM CONFERENCE
The interdisciplinary aspect and theme of the Special Session are related to the conference’s research areas on multimodal analysis, multimodal dialogue systems, visual question-answering, language reasoning, etc. using LLMs. We will have invited papers and pre- sentation as well from eminent researchers in the field.
In particular, the general theme of the Special Session is multimodal understanding that represents an interdisciplinary challenge for processing the textual, textual-visual nature of multimodal data and design systems that analyze them jointly. To this end, MLLMAis the forum that aims to bring together researchers from a variety of strongly related areas dealing with understanding cross-modal rela- tions, multimodal data processing, analysis, large language models, and their reasoning abilities.
SPECIAL SESSION TOPICS AND THEMES
The MLLMA’2025 aims to gather original and unpublished research in the domain of representation learning for multimodal data, span- ning areas such as vision and language, cross-modal learning for NLP, and enhancing the reasoning capabilities of LLMs.
The special session, MLLMA will give equal consideration to both innovative scientific methodologies and techniques for analyzing, extracting, and enriching multimodal data, as well as application- oriented perspectives. We welcome submissions of papers that are application-focused, as well as those that are more theoretical or position papers.
The topics of interest for the MLLMA’2025 include aspects related to processing multimodal data, starting with broader topics such as cross-modal learning, multimedia information processing (text/image/video), misinformation detection, multimodal representation learning. The topics of interest for the special session include but are not limited to:
- Multimodal Event Detection, and Understanding
- Multimodal News Analytics
- Multimodal Sentiment Analysis
- Multimodal Emotion Recognition
- Multimodal Sarcasm Detection
- Multimodal Hate Speech Detection
- Misinformation Detection for Multimodal Data
- Multimodal AI Content Generation
- Multimodal Behaviour Understanding
- Unsupervised, Self-supervised, or Semi-supervised Learning for Multimodal Data
- Multimodal Question Answering Systems
- Image-text Relations, Cross-modal Relations
- Semantic Relations (semiotics)
- Multimodal Rhetoric in Online Media
IMPORTANT DATES
Special Session Paper Submission: 22 Jul. 2024
Notification: 24 Sept. 2024
Camera Ready Submission: 23 Oct. 2024
Conference: 7-10 Jan. 2025
SUBMISSION
Limited to 12 content pages, including all figures, tables, and appendices, in the Springer LNCS style. Additional 2 pages containing only cited references are allowed. To submit to MMM 2025, please go to the MMM 2025 conftool submission site. https://www.conftool.pro/mmm2025/ .
PROGRAM
We will announce later
ORGANIZING COMMITTEE
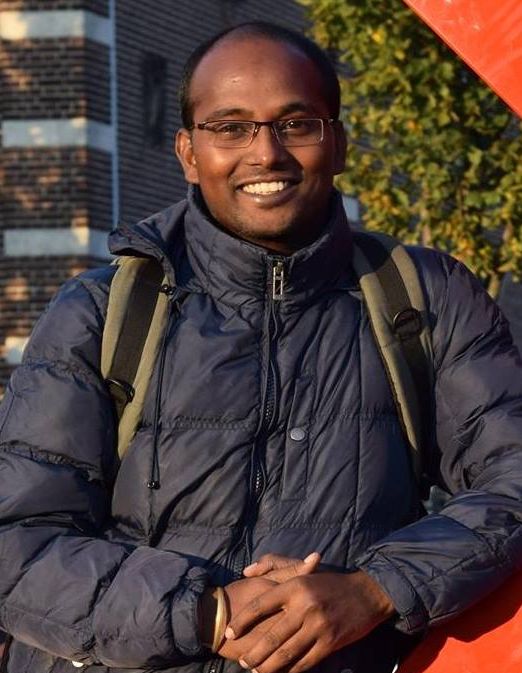
Rajiv Ratn Shah, Indraprastha Institute of Information Technology, Delhi, India. Rajiv Ratn Shah currently works as an Assistant Professor in the Department of Computer Science and Engineering (joint appointment with the Department of Human-centered Design) at IIIT-Delhi. Before joining IIIT-Delhi, he worked as a Research Fellow in Living Analytics Research Center (LARC) at the Singapore Management University, Singapore. Dr. Shah is the recipient of several awards, including the prestigious Heidelberg Laureate Forum (HLF) and European Research Consortium for Informatics and Mathematics (ERCIM) fellowships. He has also received the best paper award in the IWGS workshop at the ACM SIG SPATIAL conference 2016, San Francisco, USA and was runner-up in the Grand Challenge competition of ACM International Conference on Multimedia 2015, Brisbane, Austraila. He is involved in organizing and reviewing of many top-tier international conferences and journals. Recently, he has organized a workshop on Multimodal Representation, Retrieval, and Analysis of Multimedia Content (MR2AMC) in the conjunction of the first IEEE MIPR 2018 conference. His research interests include multimedia content processing, natural language processing, image processing, multimodal computing, data science, social media computing, and the internet of things.
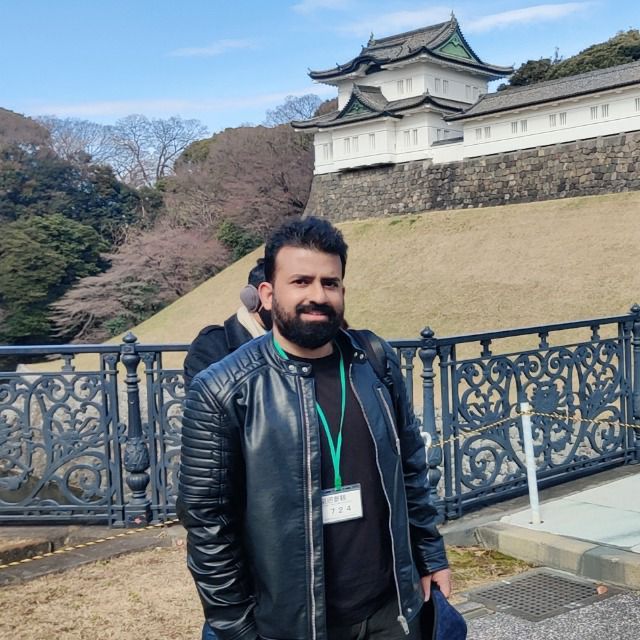
Avinash Anand, PhD, Indraprastha Institute of Information Technology, Delhi. Avinash Anand is a PhD student at IIIT Delhi and University of Buffalo, USA. He is also an Overseas Research Fellow at IIIT Delhi and pursuing his internship at NUS, Singapore under the supervision of Prof Roger Zimmermann.
Yaman Kumar, PhD, Indraprastha Institute of Information Technology, Delhi, Research Scientist-2, Adobe. Yaman Kumar is a PhD student at IIIT Delhi and University of Buffalo, USA. He is also a Google PhD fellow. Prior to joining his PhD program, Yaman had done his BTech from NSIT, Delhi. Currently, he is also working with Adobe systems as a Research Scientist. He is also the recipient of several awards at top conferences such as the best student paper award at AAAI 2019. His EMNLP 2023 paper was also selected as one of the best paper candidates.
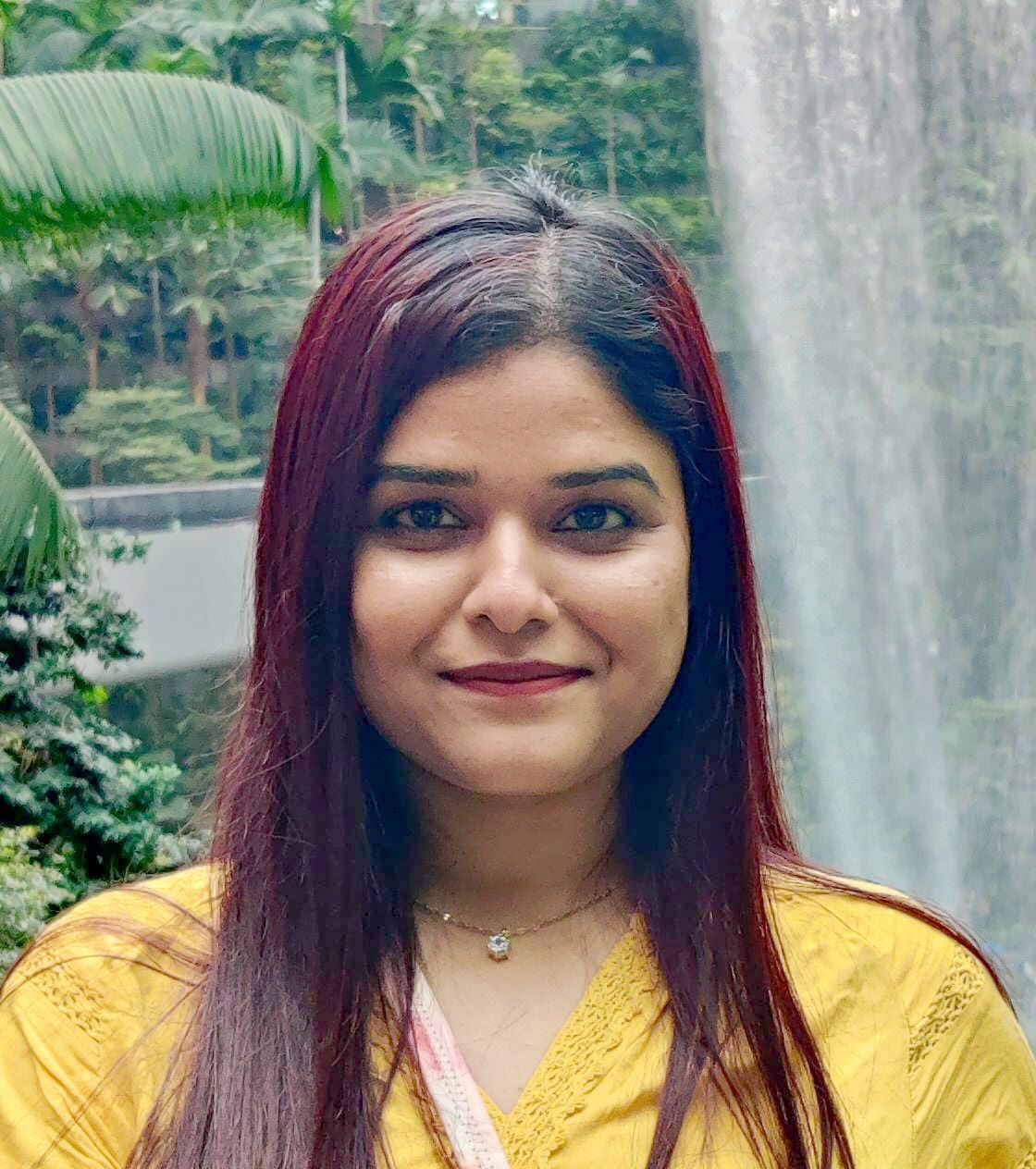
Astha Verma, Post Doc, National University of Singapore. Astha Verma has completed her PhD from IIIT Delhi. She is pursuing her postdoc at NUS, Singapore under the supervision of Prof Atrey Kankanhalli.
PROGRAM COMMITTEE
Each submission will be reviewed by at least three members of the Program Committee (PC). Papers will be evaluated according to their significance, originality, technical content, style, clarity, and relevance to the Special Session. The PC consists of well-recognized experts in the areas of NLP, Computer Vision, Multimodal Analytics, Information Retrieval, and Large Language Models. A preliminary list of PC members, 50% of whom have confirmed their interest, is as follows:
- V. Raghava Mutharaju, Indraprastha Institute of Information Technology, Delhi Google scholar
- Jainendra Shukla, Indraprastha Institute of Information Technology, Delhi Google scholar
- Roger Zimmermann, NUS, Singapore Google scholar
- Dinesh Kumar Vishwakarma, IIIT Delhi, India Google scholar
- Mukesh Prasad, UTS, Australia Google scholar
- Yi Yu, NII, Japan Google scholar
- Manoranjan Mohanty, UTS, Australia Google scholar
- Hitkul TCS PhD Fellow, Google Scholar
- Yaman K Singla,Google PhD Fellow,Prime Minister PhD Fellow,Joint PhD student with the State University of New York at Buffalo, Research Scientist in Adobe MDSR, Google Scholar
- Mohit Sharma,Microsoft PhD Fellow,
Google Scholar- Hemant Yadav,Microsoft PhD Fellow,
Google Scholar - Yaman K Singla,Google PhD Fellow,Prime Minister PhD Fellow,Joint PhD student with the State University of New York at Buffalo, Research Scientist in Adobe MDSR, Google Scholar
REFERENCES
- Valentina Aparicio, Daniel Gordon, Sebastian G. Huayamares, and Yuhuai Luo. (2024). BioFinBERT: Finetuning Large Language Models (LLMs) to Analyze Sentiment of Press Releases and Financial Text Around Inflection Points of Biotech Stocks. arXiv:2401.11011 [q-fin.GN]
- Taraneh Ghandi, Hamidreza Pourreza, and Hamidreza Mahyar. (2023). Deep Learning Approaches on Image Captioning: A Review. Comput. Surveys 56(3), 1–39. https://doi.org/10.1145/3617592
- Songning Lai, Xifeng Hu, Haoxuan Xu, Zhaoxia Ren, and Zhi Liu. (2023). Multi-modal Sentiment Analysis: A Survey. arXiv:2305.07611 [cs.CL]
- Xinwei Long, Jiali Zeng, Fandong Meng, Zhiyuan Ma, Kaiyan Zhang, Bowen Zhou, and Jie Zhou. (2024). Generative Multi-Modal Knowledge Retrieval with Large Language Models. arXiv:2401.08206 [cs.IR]
- Bertalan Mesko. (2023). The Impact of Multimodal Large Language Models on Healthcare’s Future (Preprint). Journal of Medical Internet Research 25(09). https://doi.org/10.2196/52865
- Zhiliang Peng, Wenhui Wang, Li Dong, Yaru Hao, Shaohan Huang, Shuming Ma, and Furu Wei. (2023). Kosmos-2: Grounding Multimodal Large Language Models to the World. arXiv:2306.14824 [cs.CL]
- Mai A. Shaaban, Abbas Akkasi, Adnan Khan, Majid Komeili, and Mohammad Yaqub. (2023). Fine-Tuned Large Language Models for Symptom Recognition from Spanish Clinical Text. https://doi.org/10.5281/ZENODO.10104138
- Paul F. Simmering and Paavo Huoviala. (2023). Large language models for aspect-based sentiment analysis. arXiv:2310.18025 [cs.CL]
- Weizhi Wang, Khalil Mrini, Linjie Yang, Sateesh Kumar, Yu Tian, Xifeng Yan, and Heng Wang. (2024). Finetuned Multimodal Language Models Are High-Quality Image-Text Data Filters. arXiv:2403.02677 [cs.CV]
- Junchao Wu, Shu Yang, Runzhe Zhan, Yulin Yuan, Derek F. Wong, and Lidia S. Chao. (2023). A Survey on LLM-generated Text Detection: Necessity, Methods, and Future Directions. arXiv:2310.14724 [cs.CL]
- Qinghao Ye, Haiyang Xu, Guohai Xu, Jiabo Ye, Ming Yan, Yiyang Zhou, Junyang Wang, Anwen Hu, Pengcheng Shi, Yaya Shi, Chenliang Li, Yuanhong Xu, Hehong Chen, Junfeng Tian, Qian Qi, Ji Zhang, and Fei Huang. (2023). mPLUG-Owl: Modularization Empowers Large Language Models with Multimodality. arXiv:2304.14178 [cs.CL]
- Zhen Zhang, Yuhua Zhao, Hang Gao, and Mengting Hu. (2024). LinkNER: Linking Local Named Entity Recognition Models to Large Language Models using Uncertainty. arXiv:2402.10573 [cs.CL]
- Wayne Xin Zhao, Kun Zhou, Junyi Li, Tianyi Tang, Xiaolei Wang, Yupeng Hou, Yingqian Min, Beichen Zhang, Junjie Zhang, Zican Dong, Yifan Du, Chen Yang, Yushuo Chen, Zhipeng Chen, Jinhao Jiang, Ruiyang Ren, Yifan Li, Xinyu Tang, Zikang Liu, Peiyu Liu, Jian-Yun Nie, and Ji-Rong Wen. (2023). A Survey of Large Language Models. arXiv:2303.18223 [cs.CL]